The quality and governance of data has never been more critical than it is today.Ā
In the rapidly evolving landscape of business technology, advanced analytics and generative AI have emerged as game-changers, promising unprecedented insights and efficiencies. However, as these technologies become more sophisticated, the adage GIGO or āgarbage in, garbage outā has never been more relevant. For data and IT professionals, understanding the critical role of data quality in these applications is not just importantāitās imperative for success.
Going Beyond Data Processing
Advanced analytics and generative AI donāt just process data; they amplify its value. This amplification can be a double-edged sword:
Insight Magnification: High-quality data leads to sharper insights, more accurate predictions, and more reliable AI-generated content.
Error Propagation: Poor quality data can lead to compounded errors, misleading insights, and potentially harmful AI outputs.
These technologies act as powerful lensesāmagnifying both the strengths and weaknesses of your data. As the complexity of models increases, so does their sensitivity to data quality issues.
Effective Data Governance is Mandatory
Implementing robust data governance practices is equally important. Governance today is not just a regulatory checkboxāitās a fundamental requirement for harnessing the full potential of these advanced technologies while mitigating associated risks.
As organizations rush to adopt advanced analytics and generative AI, thereās a growing realization that effective data governance is not a hindrance to innovation, but rather an enabler.
Data Reliability at Scale: Advanced analytics and AI models require vast amounts of data. Without proper governance, the reliability of these datasets becomes questionable, potentially leading to flawed insights.
Ethical AI Deployment: Generative AI in particular raises significant ethical concerns. Strong governance frameworks are essential for ensuring that AI systems are developed and deployed responsibly, with proper oversight and accountability.
Regulatory Compliance: As regulations like GDPR, CCPA, and industry-specific mandates evolve to address AI and advanced analytics, robust data governance becomes crucial for maintaining compliance and avoiding hefty penalties.
But despite the vast mines of information, many organizations still struggle with misconceptions that hinder their ability to harness the full potential of their data assets.Ā
As data and technology leaders navigate the complex landscape of data management, itās crucial to dispel these myths and focus on strategies that truly drive value.Ā
For example, Gartner offers insights into the governance practices organizations typically follow, versus what they actually need:
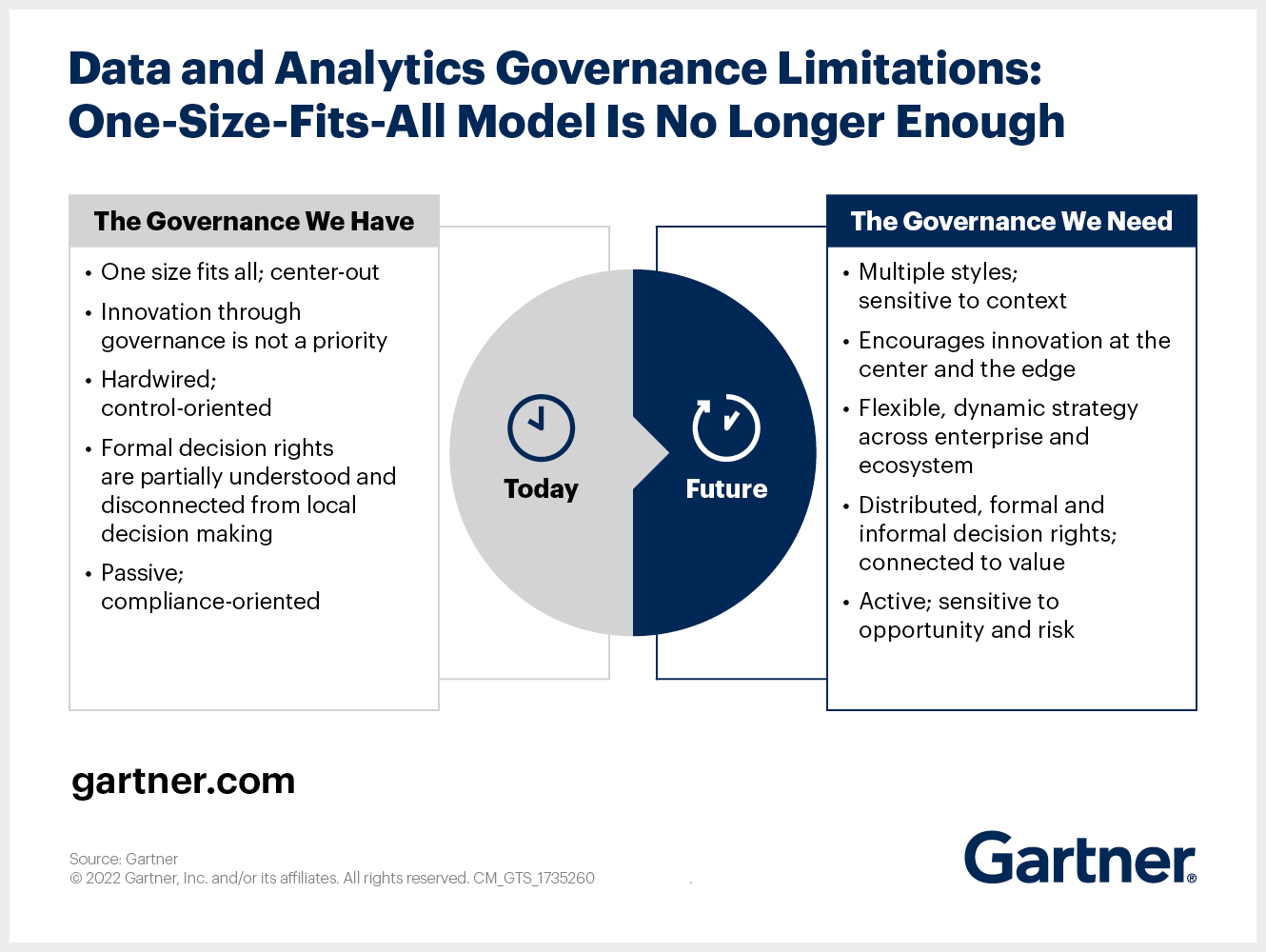
Source: Gartner
5 Data Myths Impacting Dataās Value
Here are five common misconceptions about data quality and governance, and why addressing them is essential.
Misconception 1: The āSet It and Forget Itā Fallacy
Many leaders believe that implementing a data governance framework is a one-time effort. They invest heavily in initial setup but fail to recognize that data governance is an ongoing process that requires continuous attention and refinement mapped to data and analytics outcomes.Ā
In reality, effective data governance is dynamic. As business needs evolve and new data sources emerge, governance practices must adapt. Successful organizations treat data governance as a living system, regularly reviewing and updating policies, procedures, and technologies to ensure they remain relevant and effective for all stakeholders.Ā
Action: Establish a quarterly review process for your data governance framework, involving key stakeholders from across the organization to ensure it remains aligned with business objectives and technological advancements.
Misconception 2: The āTechnology Will Save Usā Trap
Thereās a pervasive belief that investing in the latest data quality tools and technologies will automatically solve all data-related problems. While technology is undoubtedly crucial, itās not a silver bullet.
The truth is, technology is only as good as the people and processes behind it. Without a strong data culture and well-defined processes, even the most advanced tools will fall short. Successful data quality and governance initiatives require a holistic approach that balances technology with human expertise and organizational alignment.
Action: Before investing in new data quality and governance tools, conduct a comprehensive assessment of your organizationās data culture and processes. Identify areas where technology can enhance existing strengths rather than trying to use it as a universal fix.
Misconception 3:. The āPerfect Dataā Mirage
Some leaders strive for perfect data quality across all datasets, believing that anything less is unacceptable. This pursuit of perfection can lead to analysis paralysis and a significant resource drain.
In practice, not all data needs to be perfect. The key is to identify which data elements are critical for decision-making and business operations, and focus quality efforts there. For less critical data, āgood enoughā quality that meets specific use case requirements may suffice.
Action: Conduct a data criticality assessment to prioritize your data assets. Develop tiered quality standards based on the importance and impact of different data elements on your business objectives.
Misconception 4: The āCompliance is Enoughā Complacency
With increasing regulatory pressures, some organizations view data governance primarily through the lens of compliance. They believe that meeting regulatory requirements is sufficient for good data governance.
However, true data governance goes beyond compliance. While meeting regulatory standards is crucial, effective governance should also focus on unlocking business value, improving decision-making, and fostering innovation. Compliance should be seen as a baseline, not the end goal.
Action: Expand your data governance objectives beyond compliance. Identify specific business outcomes that improved data quality and governance can drive, such as enhanced customer experienced or more accurate financial forecasting.
Misconception 5: The āIT Departmentās Problemā Delusion
Thereās a common misconception that data quality and governance are solely the responsibility of the IT department or application owners. This siloed approach often leads to disconnects between data management efforts and business needs.
Effective data quality and governance require organization-wide commitment and collaboration. While IT plays a crucial role, business units must be actively involved in defining data quality standards, identifying critical data elements, and ensuring that governance practices align with business objectives.
Action: Establish a cross-functional data governance committee that includes representatives from IT, business units, and executive leadership. This committee should meet regularly to align data initiatives with business strategy and ensure shared responsibility for data quality.
Move From Data Myths to Data Outcomes
As we approach the complexities of data management in 2025, itās crucial for data and technology leaders to move beyond these misconceptions. By recognizing that data quality and governance are ongoing, collaborative efforts that require a balance of technology, process, and culture, organizations can unlock the true value of their data assets.
The goal isnāt data perfection, but rather continuous improvement and alignment with business objectives. By addressing these misconceptions head-on, data and technology leaders can position their organizations for success in an increasingly competitive world.
The post 5 Misconceptions About Data Quality and Governance appeared first on Actian.