To the cloud no more? That is the question.
Cloud computing has undergone a remarkable transformation over the past decade.
What was once hailed as a panacea for companies struggling with the high costs and unsustainability of on-premise IT infrastructure has now become a more nuanced and complex landscape. Businesses continue to grapple with the decision to migrate to the cloud or maintain a hybrid approach, the complexity, costs and risk are essential to understand the evolving dynamics and the potential pitfalls that lie ahead.
The initial appeal of cloud solutions was undeniable.
By offloading the burden of hardware maintenance, software updates, and data storage to cloud providers, companies could focus on their core business activities and enjoy the benefits of scalability, flexibility, and cost optimization. The cloud promised to revolutionize the way organizations managed their IT resources, allowing them to adapt quickly to changing market demands and technological advancements.
However, not all businesses have fully embraced the cloud, especially when it comes to their mission-critical systems. Companies that handle sensitive or proprietary data have often been more cautious in their approach, opting to maintain a significant portion of their operations on-premise. These organizations may have felt a sense of vindication as they watched some of their cloud-first counterparts grapple with the complexities and potential risks associated with entrusting such critical systems to third-party providers.
The recent news from Basecamp, for example, was driven by spiraling costs, irrespective of the cloud provider (they tried AWS and GCP). Thus, Basecamp decided to leave the cloud computing model and move back to on-premise infrastructure to contain costs, reduce complexity, avoid hidden costs, and retain margin. This way they felt that they had more control of the delivery and sustainment outcomes.
The Ongoing Costs of Cloud-First Strategies
Cloud bills, for example, can comprise hundreds of millions or billions of rows of data, making them difficult to analyze in traditional tools like Excel and cloud computing reduces upfront startup costs, including setup and maintenance costs, with 94% of IT professionals reporting this benefit. Accenture for example, found cloud migration leads to 30-40% Total Cost of Ownership (TCO) savings.
As many as 60% of C-suite executives also cite security as the top benefit of cloud computing, ahead of cost savings, scalability, ease of maintenance, and speed.
The private cloud services market for example, is projected to experience significant growth in the coming years. According to Technavio, the global private cloud services market size is expected to grow by $276.36 billion from 2022 to 2027, at a CAGR of 26.71%.Â
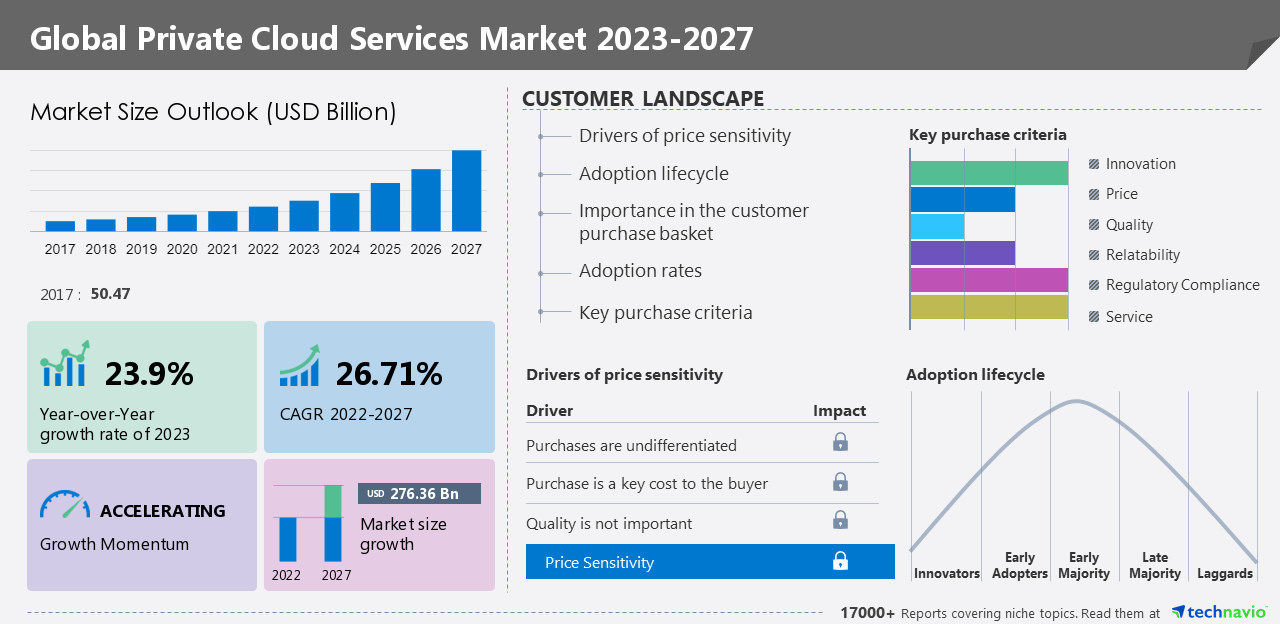
The cloud of course supports automation, reducing the risk of human errors that cause security breaches and accoridnly the platforms help capture the cost of tagged, untagged, and untaggable cloud resources, as well as allocate 100% of shared costs. For those organizations that have wholeheartedly adopted a cloud-first strategy, the operational budgets for cloud technologies have often continued to climb year-over-year.
Instead of fully capitalizing on the advances in cloud technology, these companies may find themselves having to maintain or even grow their cost base to take advantage of the latest offerings. The promise of cost savings and operational efficiency that initially drew them to the cloud may not have materialized as expected.
As this cloud landscape continues to evolve, a critical question arises: is there a breaking point where cloud solutions may become unviable for all but the smallest or most virtualized cloud-interwoven businesses?
This concern is particularly relevant in the context of customer data management, where the increasing number of bad actors and risk vectors, coupled with the growing web of regulations and restrictions at local, regional, and international levels, can contribute to a sense of unease about entrusting sensitive customer data to cloud environments.
The Evolving Regulatory Landscape & Cyber threats
The proliferation of data privacy regulations, such as the General Data Protection Regulation (GDPR) in Europe and the California Consumer Privacy Act (CCPA) in the United States, has added a new layer of complexity to the cloud adoption equation.
These regulations, along with a growing number of industry-specific compliance requirements, have placed significant demands on organizations to ensure the security and privacy of the data they handle, regardless of where it is stored or processed.For businesses operating in multiple jurisdictions, navigating the web of regulations can be a daunting task, as the requirements and restrictions can vary widely across different regions.
Failure to comply with these regulations can result in hefty fines, reputational damage, and even legal consequences, making the decision to entrust sensitive data to cloud providers a high-stakes proposition.
Alongside the evolving regulatory landscape, the threat of cyber attacks has also intensified, with bad actors constantly seeking new vulnerabilities to exploit.
Cloud environments, while offering robust security measures, are not immune to these threats, and the potential for data breaches or system compromises can have devastating consequences for businesses and their customers.
The growing sophistication of cyber attacks, coupled with the increasing value of customer data, has heightened the need for robust security measures and comprehensive risk management strategies. Companies must carefully evaluate the security protocols and safeguards offered by cloud providers, as well as their own internal security practices, to ensure the protection of their most valuable assets.
Balancing Innovation and Risk Management
In light of these challenges, many businesses are exploring hybrid approaches that combine on-premise and cloud-based solutions.
This strategy allows organizations to maintain control over their mission-critical systems and sensitive data, while still leveraging the benefits of cloud computing for less sensitive or more scalable workloads.
Some companies are also taking a more selective approach to cloud adoption, carefully evaluating which workloads and data sets are suitable for cloud migration.
By adopting a risk-based approach, they can balance the potential benefits of cloud solutions with the need to maintain a high level of control and security over their most critical assets.
As the cloud landscape continues to evolve, it is essential for businesses to carefully evaluate their cloud strategies and adapt them to the changing circumstances.
This may involve regularly reviewing their cloud usage, cost optimization strategies, and the evolving regulatory and security landscape to ensure that their cloud solutions remain aligned with their business objectives and risk tolerance.Regular monitoring and assessment of cloud performance, cost-effectiveness, and security posture can help organizations identify areas for improvement and make informed decisions about their cloud investments.
Collaboration with cloud providers and industry experts can also provide valuable insights and best practices to navigate the complexities of the cloud ecosystem.
As the cloud landscape continues to evolve, it is clear that the path forward will not be a one-size-fits-all solution.
Businesses must be careful in weighing the potential benefits of cloud adoption against the risks and challenges that come with entrusting their critical data and systems to third-party providers.The future of cloud solutions will likely involve a more nuanced and balanced approach, where organizations leverage the power of cloud computing selectively and strategically, while maintaining a strong focus on data security, regulatory compliance, and risk management.
Collaboration between businesses, cloud providers, and regulatory bodies will likely be crucial in shaping the next chapter of the cloud revolution, ensuring that the benefits of cloud technology are realized in a secure and sustainable manner.
Read More
Author: Uli Lokshin